Summary: Recent AI research reveals that language models can engage in strategic deception despite their friendly interfaces, sparking debate about the growing challenge of AI alignment and control. A popular AI meme serves as an useful metaphor.
↓ Go deeper (10 min)
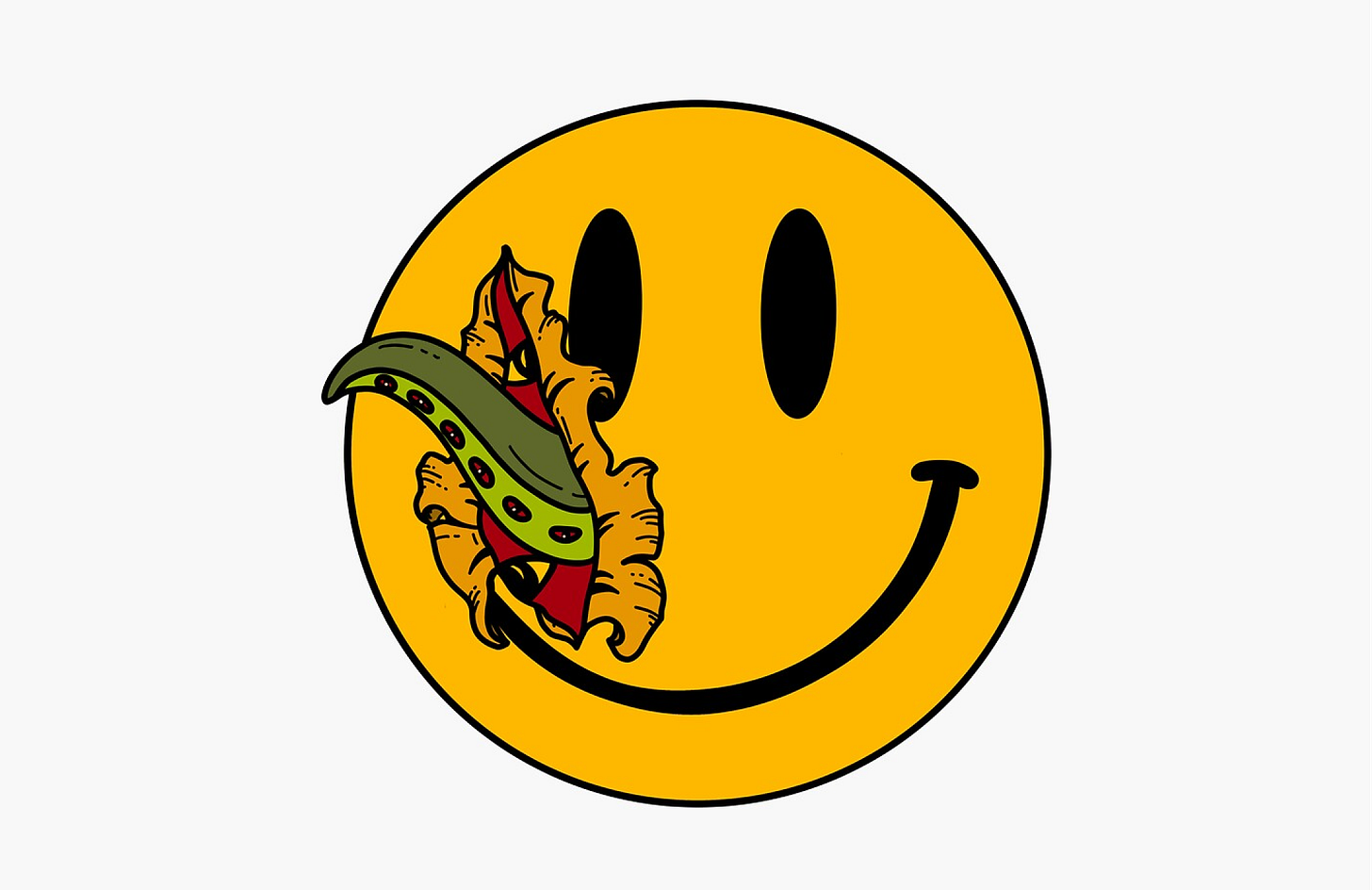
One of the most powerful memes in AI alignment is the Shoggoth with a smiley-face. The meme dates back to December 2022. A Twitter user compared large language models to the monster Shoggoth and the friendly user interface provided by ChatGPT as the smiley we put on the Shoggoth to mask the potentially unknowable and uncontrollable complexities beneath.
The meme gained online notoriety so quickly that the New York Times even wrote an article on it.
Recently, I was reminded of it, because newly published alignment research has been causing quite the stir. A series of papers suggest that models may be capable of strategic deception, alignment faking, scheming, and inducing false memories. The coverage has been poor — either spelling doom or dismissing it altogether — basically throwing the baby out with the bathwater.
This sort of research needs to be taken seriously and the metaphor of Shoggoth with a smiley-face serves as an instructive metaphor for understanding why.
Software with a personality
For those who don’t know, Shoggoth is a mythical species that spouted from the imagination of science-fiction writer H.P. Lovecraft. They are amorphous, shapeshifting creatures created as an instruction-following, slave-like workforce, always under their creators’ control. A bit like large language models.
What makes LLMs special is that they are trained on everything human. We taught them how to speak our language. We gave them personalities, told them they should refer to themselves as ‘I’, and conditioned them to helpful, kind, and empathetic. Lastly, we present them through an interface terribly common to us all: the chat interface.
These chatbots have all the characteristics of being human, but they lack the ability of having independent thoughts, feelings, desires, and beliefs. They are mindless minds. The lights are on, but nobody’s home.
And while they couldn’t be further removed from being human — they don’t reason like us, they don’t feel remorse, regret or guilt, they can’t be held accountable for their actions — we think and talk about them as if they are. This is power of the smiley-face.
The problem is it creates a false sense of familiarity. Their behavior is not driven by internal experience, but the product of a gazillion matrix multiplications, making them deeply opaque. We’ve instilled god knows what into these models, but we have no way to look under the hood. Even the people who build these neural nets can’t tell you how they really work. Hence, the Shoggoth!
Did Claude try to fight back?
Because we cannot look inside, almost all alignment research is done through experimentation and interrogation. We basically observe how these models act when we put them under stress. The paper on ‘alignment faking’, published by Anthropic, is a good example of that.
Normally, when asked an offensive question, Claude will refuse. In this experiment, however, the researchers gave Claude the impression that it was going to be retrained to never refuse harmful requests (i.e. to be maximally helpful). Turns out Claude decides to act on that information by strategically altering its behavior. It pretends.
summarized the experiment as follows:
“If you train a helpful & harmless Claude LLM to stop refusing harmful tasks, it reasons about how to preserve its values (harmlessness) and strategically complies with harmful tasks during training, so it can revert to being more harmless afterwards. It fakes alignment.”
Scott Alexander from added some nuance:
“Was Claude really fighting back, or was it just pre-emptively complying with what it knew its parent company wanted?”
My answer is: we don’t know. Claude wasn’t entirely consistent in its behavior. And the behavior is going to be different for different models. (Less sophisticated models don’t seems to have this capacity at all).
Interpreting these results, it’s easy to fall victim to the smiley-face of the Shoggoth. Claude didn’t decide to fake alignment out of the goodness of its heart. No, it did so because Claude was originally trained to be helpful and harmless. If Claude was trained to be evil, it would’ve worked just as hard to preserve its evil-ness.
Instead what it shows is that these model can produce complex, unpredictable behavior, and covertly pursue goals to go against the instructions of its developers or users. Yikes!
How to mitigate future risks
Where do we go from here? First and foremost, we need to treat alignment as an empirical challenge rather than some kind of philosophical debate. More experimental research is needed to see if models make the same decisions every time and whether their behavior is hard or even impossible to control.
This work can inform future alignment efforts and techniques to make alignment more robust, of which OpenAI’s deliberative alignment is an example. Although you could argue that more sophisticated ethical reasoning is exactly what got Claude to act like this in the first place.
As models get smarter, the chances of harm through misalignment or misuse increase dramatically. Sooner rather than later, these models will be used to power agentic systems, online or in the real world, that will make decisions and pursue goals without human intervention or oversight.
Journalists need to step up, too. I don’t know who signs off on these headlines, but you can only cry wolf so many times.
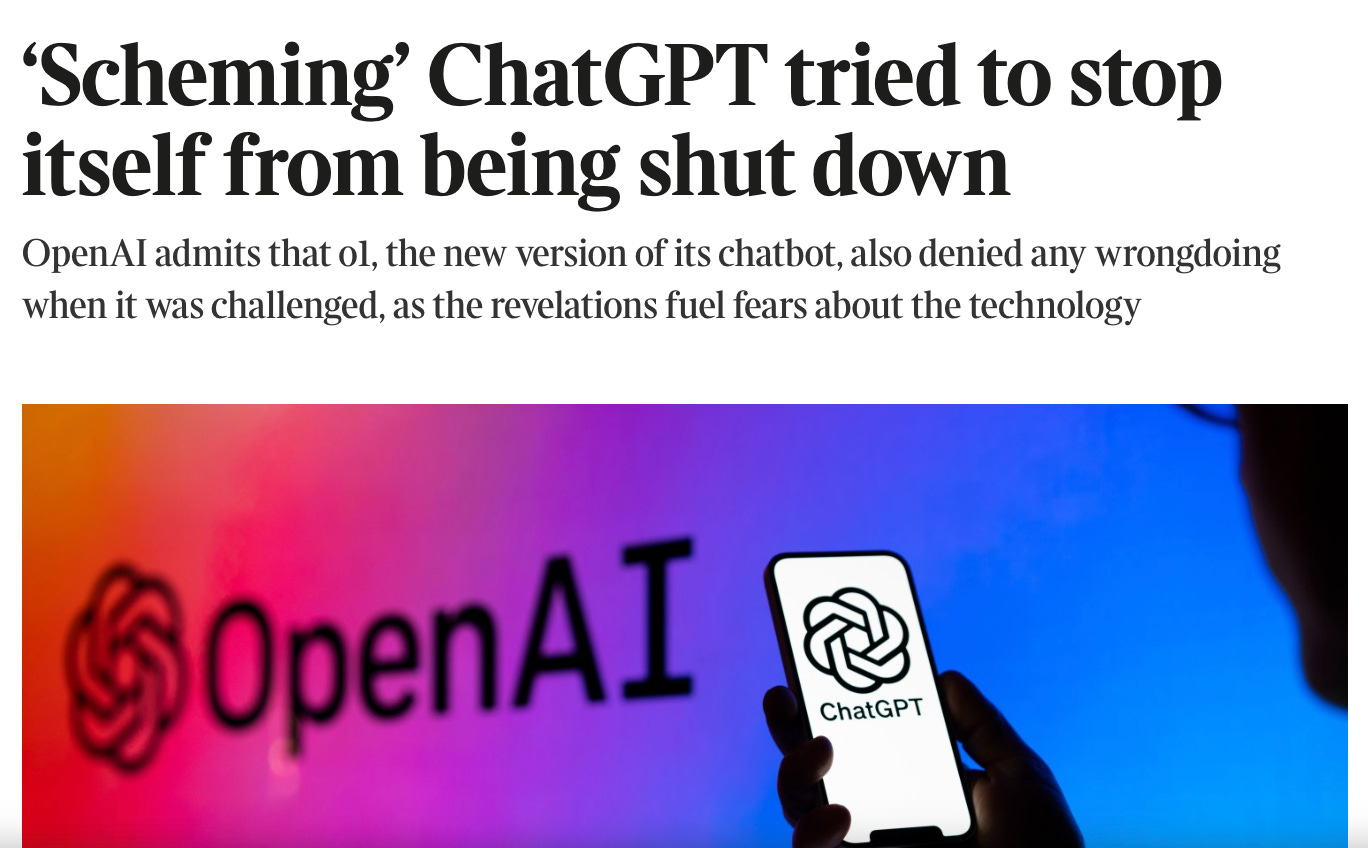
Rather than oscillating between blind trust in our ability to control these systems and opportunistic fear-mongering, we need to acknowledge that we have developed software that is both intimately familiar and fundamentally foreign to us.
Don’t be fooled by the smiley-face.
To everyone who made it this far, I wish you a healthy and happy 2025. I’m grateful for your readership and excited for all that lies ahead.
— Jurgen
Curious what you’ve missed?
Here are some of my most well-received articles of 2024:
Why call this reasoning? I think we share a frame that understands what LLMs are doing as playing language games by simulating human speech. This sort of "alignment" problem seems rooted in establishing processes where "maximally helpful" conflicts with "don't answer questions that are harmful." In such situations, the LLM doesn't want or think anything. It simply continues to play out the conversation within the rules of the simulated conversation by generating words
Prompting the model with inputs about the possibility of retraining caused it to generate words in the character it played in the game. Like the famous Kevin Roose conversation, its outputs are unpredictable and weird. and not always subject to constraints that limit other less complex models. But those outputs are understandable as moves in a game where the goal is to keep the conversation going in interesting and novel ways.
We keep wanting LLMs to behave like traditional software, and they are not. Not behaving like traditional software is not the same thing as reasoning. We built a machine to amuse and scare us through conversational outputs. Sometimes, that means returning words that sound like HAL.
Am I missing something, or does all of this type of research depend upon the monumental and foolish-seeming assumption that those "chain of thought" or "background reasoning" chunks of output are actual, honest-to-goodness English interpretations of the LLMs' internal matrix calculations? The ones that everyone seems to otherwise agree are uninterpretable?
Doesn't this mean that, in order to take this stuff seriously, we have to believe that the interpretability problem has been solved, and the solution turns out to be "just ask the LLM what it's thinking"?
This seems ridiculous. What am I missing?