Key insights of today’s newsletter:
The ‘Reversal Curse’ refers to a 2023 study that showed that large language models that learn “A is B” don’t automatically generalize “B is A”.
A recent pre-print paper that focuses on medical Visual Question Answering (MedVQA) suggest this phenomenon also transfers to multimodal models. Uh-oh!
While these models continue to shatter records on industry benchmarks, the researchers call into question the robustness of these evals: what are they even measuring?
↓ Go deeper (8 min read)
In September 2023, researchers showed that large language models suffer from a phenomenon called the ‘Reversal Curse’. They demonstrated that if a model has learned “A is B”, it won’t necessarily generalize to the reverse direction “B is A”. One of the viral examples was Who is Tom Cruise’s mother? Initially, GPT-4 would answer this question correctly, however, when posed the reversal question Who is Mary Lee Pfeiffer’s son?, it would fail.
Last week, a new preprint paper Worse than Random? An Embarrassingly Simple Probing Evaluation of Large Multimodal Models in Medical VQA was published, which suggests that GPT-4 Vision suffers from the same ails and the reversal curse also transfers to multimodal models.
So, buckle up and get ready for the sequel: The Reversal Curse Returns!
Embarrassingly simple
In medical Visual Question Answering (MedVQA) benchmarks, multimodal models have shown remarkable gains over the past year. The results of this study, however, seriously call into question the reliability of these evaluations.
When subjected to robust probing, including testing for negations, the performance drop is… shocking. All models suddenly perform worse than random guessing.
The embarrassing part is how simple the probing is. Similar to the 2023 study, the researchers tested a series of questions and their ‘adversarial pair’. Here’s an example of what that looks like:
While the models can be accurate in one way, when tested for the negation, they often fail to produce the right answer.
These findings should give us pause. Not only does it expose the brittleness of these models, it also undermines the notion that they are somehow capable of generalization. This is particularly stinging because some industry leaders touted that multimodality would solve some of the challenges that models only trained on language suffer from; that it somehow would make them smarter.
On top of that, we should be asking ourselves: how is it possible that this relatively simple adversarial questioning-technique completely wipes out the performance gains these companies claim to have made on these benchmarks? The answer is simple: the benchmarks are flawed.
AI has a benchmark-problem
While AI companies are obsessed with outperforming each other on all kinds of evals, criticism on their robustness is growing.
In a recent article, Professor Melanie Mitchel debunks the claim that “AI now beats humans at basic tasks”. Reasons for why we should be skeptical of benchmarks that suggests AI has achieves human-level performance are: data contamination, over-reliance on training data, and so-called shortcut learning.
As an example, Mitchell refers to a study that showed how an AI system that was able to successfully classify malignant skin cancers from photographs was actually using the presence of a ruler in the images as the most important predictor (because photos of nonmalignant tumors were less likely to include rulers).
A giant red flag is that while AI companies are happy to publishes their scores, there’s no way for scientists to seriously scrutinize these results, because both the training methods and training data are not openly shared. It’s therefore impossible to know whether one of these things (or all of the above) are happening.
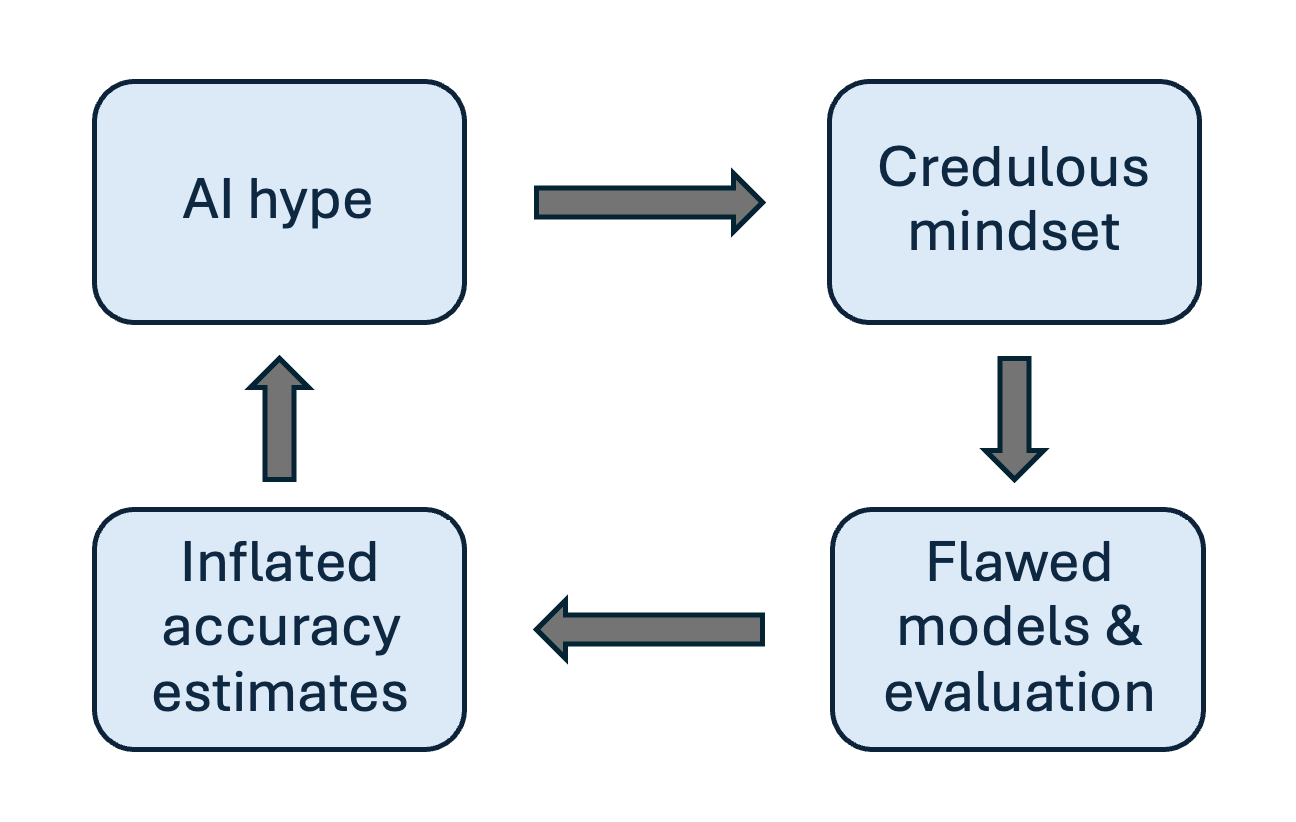
AI companies also have a strong incentive to cheat. If you want your model to do well on standardized tests, it’s very tempting to train them on a bunch of similar-looking tests. Over-attributing qualities to these models generates hype and can help a company secure a next round of funding. It’s a perverse feedback loop that runs deep; not just in business, but also in science.
The proof is in the pudding
So, the next time a company like Anthropic or OpenAI claims to have shattered a new record, we should probably take the news with a pinch of salt. Let’s not succumb to magical thinking or meaningless extrapolations that only add to the confusion about a reasonable trajectory for progress. The proof, as always, is in the pudding.
And as far as the ‘Reversal Curse’ goes, I think Andrej Karpathy, ex-OpenAI cofounder, during a one-hour talk said it best when he stated: “it seems like the knowledge in these models can only be accessed by coming at it from a certain direction.” That’s a very euphemistic way of saying: these models are unreliable.
Join the conversation 🗣
Share, like or leave a comment if it resonated with you.
This should not be an impossible problem to solve if you instantiate a reversibility check during fine-tuning stages (or RLHF stages). Even in the stages of reinforcement learning from human feedback pipelines, it should be possible to check for the presence of reversibility in a prompt as an additional attribute (in addition to "how correct is this output", add something like "is this an appropriately posed reversed question, and is the reversed instance reversible relative to the initial prompt"), instantiate the reversible output, evaluate whether the reversible instance is true or not (by finding the inverse question and answering it or something, as said before), and further train and reward with the reversible instance when it is true, reward a bit more when models correctly identify reversible instances, or something along those lines.
I wonder if solving these kinds of problems (of compositionality, more generally) will help with generalization, and if so, kudos to you for writing this post so timely.
Maybe even fields like robotics algorithms, if properly implemented and integrated into these LLM domains (including NLP and MLM), can be useful in solving this problem.
You might want to think about something along these lines: https://arxiv.org/pdf/2106.04480v2
It's almost as these are next token prediction models that are great at mimicry but have no concept of truth!
I feel a little sad that I don't get to appreciate LLMs for the amazing technical achievements that they are, because right from the off their creators and owners and cheer teams have been overhyping them to absurd levels. How I wish for a prominent figure in tech to come out and say "machine learning is great but there's no reason to think any AI is going to develop any kind of human-like cognitive ability because brains aren't statistical models and it would be best for all of us to just stop comparing AIs to minds".