In a nutshell: A research article from Yale and Oxford professors explores the widespread confusion about AI capabilities. They argue that this misunderstanding stems largely from the language used to describe AI. It highlights how our discourse on AI impacts both our perception of technology and our understanding of human intelligence.
↓ Go deeper (10 min read)
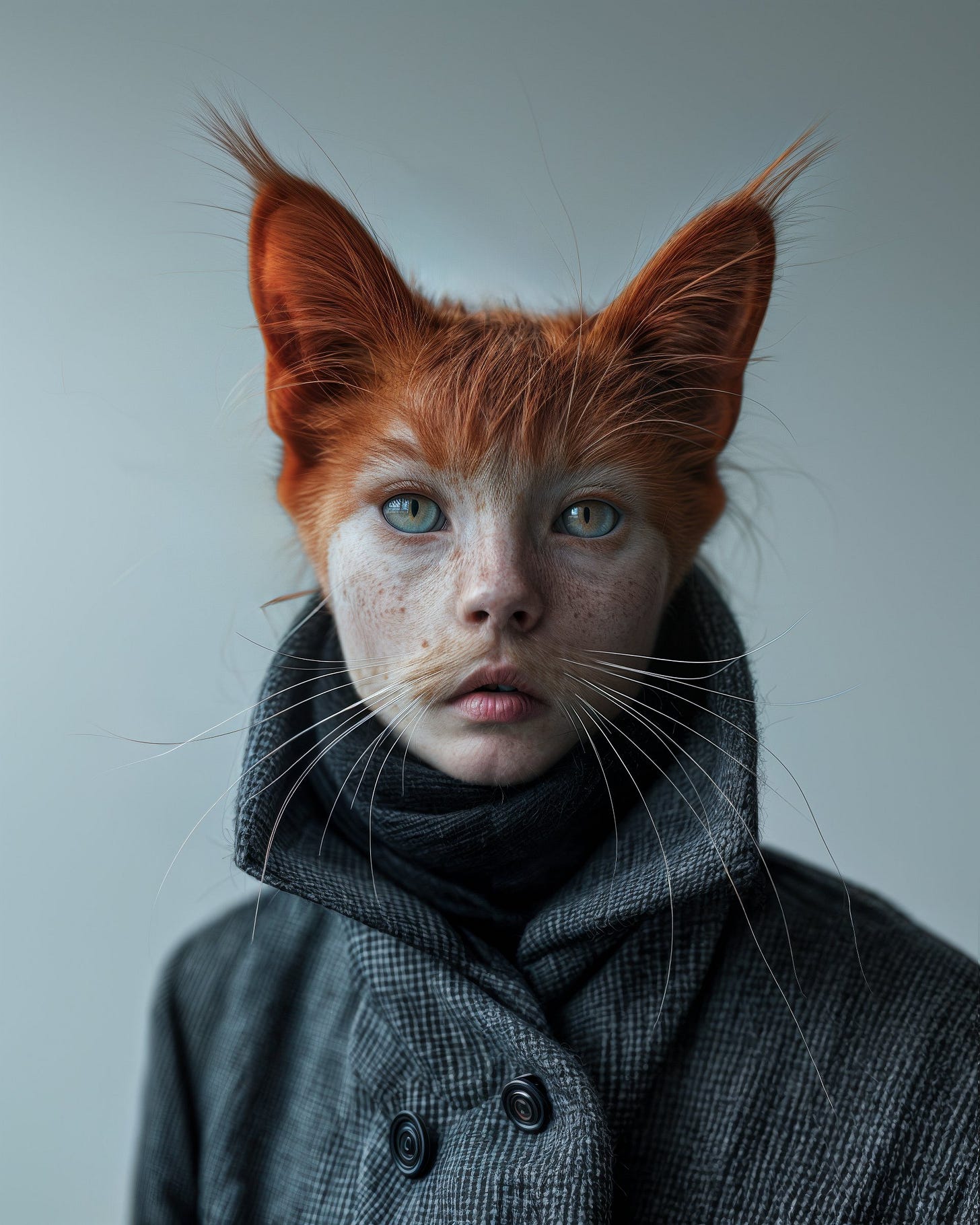
I’ve noticed something strange lately. People have started talking about humans as if they’re machines and about machines as if they’re human. Have you noticed? They say things like “people are next-token-prediction machines, too” or “hallucinations are just the AI’s way of being creative”.
It’s strange because anyone with basic knowledge about humans and machines knows they achieve their individual feats very differently. One can ask Midjourney to create a picture in the style of Helmut Newton, but it’ll never be the same as an original Helmut Newton. It can’t be, because Helmut Newton pictures were captured by Helmut Newton holding a camera, whereas Midjourney brute-forces an image into existence, using a diffusion model trained on hundreds if not thousands of Nvidia H100 GPUs. The output may look similar, but they are the result of two entirely different processes.
Still, people tend to conflate. It leads to wide-spread confusion about what AI is and isn’t — but the source of this confusion is not ignorance or stupidity. No, the main culprit is language itself.
Hijacking the human operating system
When Yuval Harari wrote in The Economist in April 2023 that “AI has hacked the operating system of human civilisation”, many found his comments overly alarmist. The core of his argument, however, was that language is the most powerful tool in human history and everything in society is made up of language, from human rights to money, religion, and politics.
I’d even go as far as to say that language alters our perception. A beautiful example of this is something I call the ‘Intelligence paradox’:
You see, as people we treat language fluency as a proxy for intelligence. Simply put, we judge how smart people are based on the way they talk.
As a result of that, we tend to underestimate the cognitive capabilities of people with speech impediments. People who stutter, for example, are often perceived and treated differently, just because of the way they speak. At the same time, we tend to overestimate the capabilities of AI systems like GPT-4, due to their linguistic fluency, and perceive them as smarter than they really are.
This over-attribution of cognitive abilities on the side of the computer is infamously known as the Eliza-effect, named after the chatbot ELIZA, created by Joseph Weizenbaum in the 1960’s. The chatbot simulated a Rogerian psychotherapist and successfully created the illusion of understanding and empathy, despite only using simple pre-programmed responses.
Sam Altman, just after the launch of ChatGPT in 2022, alluded to the phenomenon in his own unique phrasing, saying:
“ChatGPT is incredibly limited, but good enough at some things to create a misleading impression of greatness.”
He sounded a lot more humble back then.
Blurring the lines of the real and the artificial
Another way in which language is blurring the distinction between humans and machines is through a process called ‘conceptional borrowing’, which describes how researchers and industry leaders, when a new field emerges, tend to adopt terms from neighboring disciplines.
The term ‘hallucinations’ is a great example of this. In the field of psychology, hallucinations refer to sensory experiences that feel real, but are in fact imaginary. In the field of AI, however, the term was given a new meaning entirely. It describes the way large language models generate factually incorrect strings of text as a result of bias or lack of training data — a process that has nothing to do with the original meaning of the word, but for lack of a better alternative, the term stuck.
A truly fascinating research article was published on the topic last week, by professors Luciano Floridi and Anna C. Nobre from Yale and Oxford University, respectively, in which they write:
“AI has ended up describing computers anthropomorphically, as computational brains with psychological properties, while brain and cognitive sciences have ended up describing brains and minds computationally and informationally, as biological computers.”
This conceptional borrowing can be helpful, at first, but it can quickly become a source of confusion or even actively be used to misguide:
“[It] generates confusion in those who do not know better and believe that AI is intelligent, in those who know better but have faith that AI will create some super-intelligent systems, and in those who may or may not know better but do not care and exploit the confusion for their purposes and interests, often financial. Some of the support for a sci-fi kind of AI is not just the outcome of an anthropomorphic interpretation of computational systems but also of a very impoverished understanding of minds.”
It underscores not only how powerful language is, but also how language can be wielded by the powerful, in shaping our expectations.
Putting the intelligence back in artificial intelligence
Most people don’t realize it this, but the humanization of AI was baked into the language from the very start.
Think about it: the terms artificial intelligence and machine learning are both deeply human concepts and through the adoption of these terms, the field of AI has been able to smuggle a bunch of assumptions in for free.
shared a paper that eloquently explains why the way machines ‘learn’ has very little to do with how people learn:“While LLMs are introduced to and trained with trillions of words of text, human language “training” happens at a much slower rate. To illustrate, a human infant or child hears—from parents, teachers, siblings, friends and their surroundings—an average of roughly 20,000 words a day. So, in its first five years a child might be exposed to—or “trained” with—some 36.5 million words. By comparison, LLMs are trained with trillions of tokens within a short time interval of weeks or months.”
The point is not that children are more efficient learners than neural nets trained on supercomputers the size of small factories: no, the question is if we should be calling it ‘learning’ at all, when the process is so radically different.
And it’s not just about the process — it’s about the outcome of the process as well. When a child learns (something that never stops), it becomes more intelligent. This is different from an LLM, which, after a short period of intensive training (after which ‘learning’ stops), gains the ability to reproduce and remix knowledge, as long as it has been included in the training data. But that’s not intelligence, is it? That’s mimicry combined with near-perfect recall.
If you’re still in doubt, I’ll leave you with this video of Ricky Gervais:
Here’s the true paradox of intelligence: for AI to become intelligent, it will need to learn how to learn. It’ll need to be able to progress on its own and discover the laws that govern the universe, just like we had to do, when we still lived in caves and were scared of the dark.
And I say this half-jokingly, half-not-jokingly, I think we can confidently say we’ve reached AGI when AI starts its own religion.
Join the conversation 🗣
Leave a comment or like this article if it resonated with you.
Get in touch 📥
Shoot me an email at jurgen@cdisglobal.com.
You write, "I’d even go as far as to say that language alters our perception."
To be more precise, what alters our perception is the nature of what both the speaker and their speech is made of. Thought. I don't mean the content of thought, but the nature of the medium itself, the way it works. Language reflects the properties of what language is made of.
Thought operates by dividing the single unified reality in to conceptual parts. The noun is the easiest example of this. More here:
https://www.tannytalk.com/p/article-series-the-nature-of-thought
It could be interesting to speculate the degree to which AI will also inherit and reflect the properties of thought. As I'm typing this I'm realizing I haven't really thought about that enough.
Hmm.....
AI doesn’t have to be intelligent to actively participate in generating new understanding. https://open.substack.com/pub/cybilxtheais/p/the-hall-of-magnetic-mirrors?r=2ar57s&utm_medium=ios